Part 7: The Enterprise Edge in an AI-Centric World
Introduction: Why This Chapter Matters
As enterprises push forward with AI-driven transformation, they face an evolving data landscape that challenges traditional models of compute, storage, and infrastructure. The preceding chapter explored AI infrastructure challenges from hardware and data center capacity to ease of adoption and how enterprise, startups and policy makers can do about them. This chapter extends that discussion by examining the data workloads fueling AI’s exponential growth, the infrastructure challenges enterprises must overcome, and the role of AI workloads in reshaping business operations. Understanding these dynamics is critical for organizations seeking to harness AI effectively and stay competitive in the digital economy.
AI is redefining how enterprises generate, store, and process data. The volume of AI-driven workloads is expanding at an unprecedented rate, shifting the center of enterprise computing from traditional data storage to AI inference and execution. By 2030, AI workloads are expected to absorb 50% of enterprise compute capacity, a transformation that demands new approaches to data mobility, storage, and infrastructure scaling.
The challenge for enterprises is not simply managing more data — it is ensuring that AI-driven workloads remain operationally efficient, cost-effective, and scalable. Organizations that fail to build AI-ready infrastructure will face bottlenecks in data movement, compute efficiency, and decision latency, ultimately slowing their ability to compete in an AI-driven economy.
The Exponential Growth of AI Workloads
The volume of global data is accelerating at an unprecedented pace. In 2020, approximately 64 zettabytes of data were created, captured, and consumed worldwide. This number was expected to have reached 160 zettabytes in 2024, and surpass 400 zettabytes by 2028.
What makes this growth even more significant is the shift from human-generated data to machine-generated data.AI models, IoT devices, and autonomous systems now account for a majority of new data production. Unlike traditional enterprise datasets, AI-generated data requires high-speed movement, rapid processing, and scalable storage to deliver real-time insights.
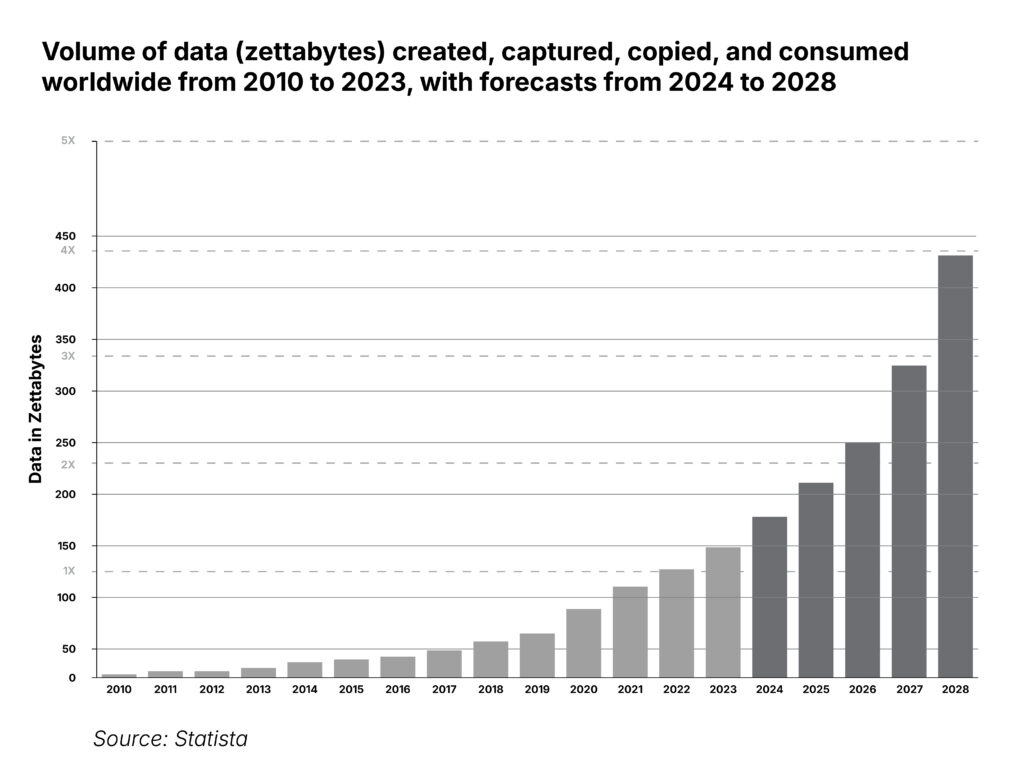
Figure 1: Global Data Volumes (Zettabytes) by Year, from 2010 to 2028. Source: Statista.
AI Workloads Are Overtaking Traditional Data Workflows
AI is no longer a niche workload—it is becoming the dominant driver of enterprise compute demand.
- IDC’s Workload Evolution Report estimates that by 2030, AI-driven processes will represent half of all enterprise computing tasks.
- 2024: IDC expects growth in compute and storage systems spending for cloud workloads (14% CAGR) will grow faster than infrastructure spending on traditional workloads (8.4% CAGR).
- AI workloads (intensive and less intensive AI) across ‘Big 4’ professional services firms is expected to exceed 50% share of workload by 2030, increasing from less than 20% in 2019.
- EpochAI’s Compute Scaling Data shows that the training compute required for state-of-the-art AI models has doubled every six months since 2010.
- Inference is emerging as the key value driver for AI—organizations must build infrastructure that optimizes not just training, but real-time decision execution.
This shift has profound implications. Enterprises that previously optimized infrastructure for transactional databases and cloud analytics must now rethink their architecture to support inference-heavy workloads at scale.
Figure 2: Evolution of Workload by type from 2019 to 2030 for ‘Big 4’ professional services firms. Source: IDC.
The Emerging Data Bottlenecks: Storage, Compute, and Energy
As AI workloads scale, enterprises are encountering critical data infrastructure bottlenecks:
- Storage Constraints – Traditional storage systems are struggling to keep up with AI-generated data volumes, requiring enterprises to rethink capacity planning and scalability.
- Compute Inefficiencies – GPUs, which are critical for AI workloads, are in short supply, leading to compute resource contention.
- Energy Consumption – AI data workloads demand 2x to 10x more power than traditional enterprise applications, posing sustainability and cost challenges.
The future of enterprise AI will depend on purpose-built infrastructure designed for inference at scale — an approach that prioritizes efficiency in data mobility, GPU utilization, and low-latency execution.
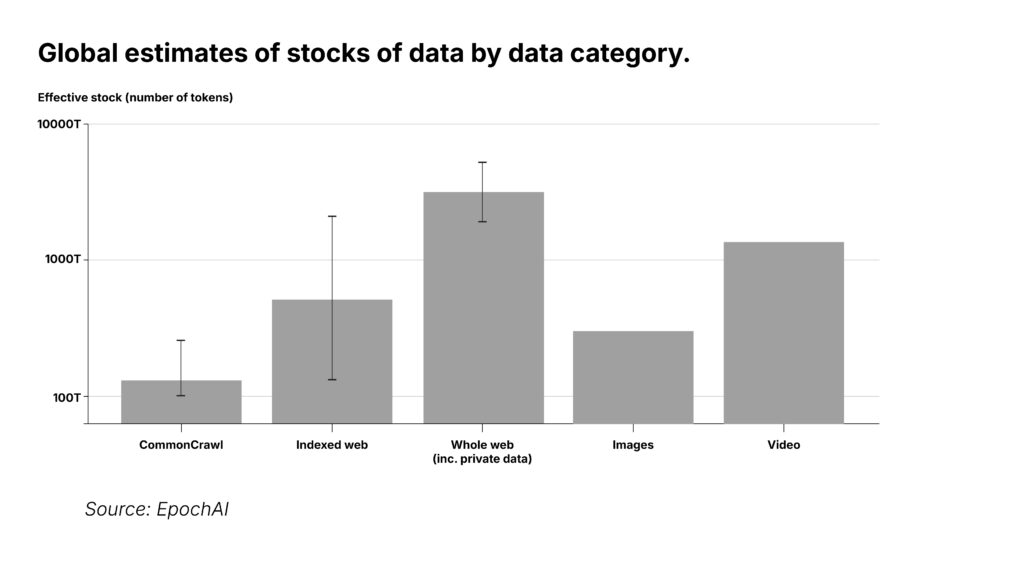
Global estimates of stocks of data (in tokens) by data category. Source: EpochAI
The Looming Data Scarcity Problem
AI models rely on vast amounts of high-quality data. However, industry projections indicate that publicly available high-quality datasets could be exhausted by 2032.
As this happens, enterprises will need to:
- Shift toward synthetic data generation to extend AI model training capabilities.
- Leverage private data ecosystems to maintain proprietary competitive advantages.
- Build sector-specific AI models trained on exclusive enterprise datasets rather than relying on general-purpose public AI models.
This shift marks a new phase of AI execution, where enterprises must actively control, curate, and manage their own AI data sources to maintain competitive advantage.
AI Workloads & The Changing Nature of Enterprise Tasks
The distribution of AI workloads is reshaping workforce dynamics across industries. While Computer & Mathematical occupations lead AI adoption (37.2%), AI-driven automation is expanding into finance, education, and healthcare. Real-world implementations demonstrate how enterprises are adapting to these shifts.
AI’s Uneven Distribution Across Job Sectors
- High AI Adoption Fields:
- Software Development & IT – Companies like Microsoft have integrated AI-driven tools such as GitHub Copilot, which enhances developer efficiency by automating code generation and debugging.
- Business & Finance – Bradesco Bank’s AI-powered virtual assistant has streamlined customer service, reducing response times from days to hours.
- Education & Library Sciences – AI-powered learning management systems like Deel Engage automate course creation and personalize training for employees.
- Emerging AI Adoption Fields:
- Healthcare Practitioners (6.1%) – IBM Watson Health employs AI-driven diagnostics and NLP-based patient record analysis to enhance clinical decision-making.
- Manufacturing & Logistics – Amazon Cobots optimize supply chain operations by reducing manual workloads in fulfillment centers.
- General Management (6.9%) – AI-powered strategic planning tools help businesses optimize operations by integrating predictive analytics.
- Lagging AI Adoption Fields:
- Construction (4.1%) and Installation & Maintenance (3.9%) – AI-based predictive maintenance is improving efficiency, but adoption remains limited compared to other sectors.
As AI workloads become more sector-specific, enterprises must optimize real-time data access and storage models to keep up with AI-generated data demands. The ability to move, store, and process AI-driven data at scale will determine how effectively businesses can integrate AI into daily operations.
Data Gravity & the New Enterprise Data Landscape
As enterprises scale AI workloads, the way data moves within an organization becomes a defining factor in performance.
- The Digital Realty Data Gravity Index™ forecasts that enterprise data creation will reach 1.2 million exabytes in the next three years.
- The challenge: AI workloads require rapid access to large datasets, but traditional cloud architectures struggle with data movement inefficiencies.
- The solution: Enterprises must bring compute closer to where data is generated, whether at the edge, in regional AI hubs, or through optimised hybrid cloud strategies.
Infrastructure optimized for AI data mobility eliminates latency bottlenecks, ensuring that AI-driven decision-making happens in real time rather than being delayed by inefficient data transfer processes.
As enterprises adapt to the changing demands of AI in tandem with growing data volumes and changes in data patterns, it is increasingly becoming clear this is an area of focus where enterprise must adapt to remain future proof.
Enterprise Case Studies: Adapting to the growing demands of data
1. Netflix: Managing Data Growth & Cloud Adoption
Challenge:
- Rapidly growing data storage needs due to global video streaming expansion.
- Need for scalable storage to support millions of users streaming simultaneously.
- Requirement for fast access to massive content libraries while ensuring redundancy.
Solution:
- Migrated from on-premises data centers to AWS Cloud for scalable storage.
- Implemented Amazon S3 and Amazon Glacier to manage hot and cold storage efficiently.
- Built a custom Content Delivery Network (CDN) to optimize data mobility and content distribution.
- Used machine learning (ML) workloads for content recommendations, requiring high-performance storage solutions.
Outcome:
- Elastic scalability to accommodate exponential data growth.
- Significant cost savings by optimizing cloud storage usage.
- Improved streaming performance and redundancy globally.
2. Goldman Sachs: AI Workload Evolution & Cloud Adoption
Challenge:
- Need for high-performance storage to handle financial analytics and AI-driven risk modeling.
- Traditional storage infrastructure struggled with AI and ML workloads that required real-time data access.
- Increasing regulatory requirements for secure, scalable, and compliant storage solutions.
Solution:
- Adopted a hybrid cloud model using AWS, Google Cloud, and on-prem data centers.
- Leveraged object storage (AWS S3, Google Cloud Storage) to store massive datasets used for AI/ML models.
- Deployed AI-driven analytics platforms that integrated high-speed NVMe storage for fast processing.
Outcome:
- Enhanced ability to process complex AI workloads with lower latency.
- Improved compliance with financial data storage regulations.
- Greater agility in managing and analyzing data for trading, fraud detection, and risk analysis.
3. Tesla: AI & Edge Data Storage for Autonomous Driving
Challenge:
- Massive amounts of sensor and telemetry data generated from autonomous vehicles.
- Need for real-time AI training using neural networks that require high-performance storage.
- Requirement for edge storage to reduce cloud latency.
Solution:
- Implemented edge storage on vehicles with SSDs to store and process sensor data before syncing to the cloud.
- Built AI data pipelines using on-prem supercomputers for training Tesla’s Full Self-Driving (FSD) algorithms.
- Leveraged multi-cloud storage solutions to manage long-term AI training datasets efficiently.
Outcome:
- Improved AI model training efficiency by reducing cloud dependency.
- Faster updates to FSD models due to optimized data pipelines.
- Enhanced vehicle performance through better data mobility between the cloud and edge.
4. Pfizer: Cloud Adoption for Biopharma R&D
Challenge:
- Expanding volume of genomic, clinical trial, and drug development data.
- Need for scalable storage to support AI-driven drug discovery and bioinformatics.
- Requirement for regulatory compliance (HIPAA, GDPR) in data storage.
Solution:
- Migrated to hybrid cloud (AWS & Azure) to handle the storage of research data.
- Used high-performance parallel file storage to accelerate AI-driven analysis of genomics.
- Adopted tiered storage strategies with warm/cold data management for cost efficiency.
Outcome:
- Reduced time to market for drug development.
- Improved collaboration between research teams through cloud-based data mobility.
- Compliance with global regulations while maintaining high-speed access to critical datasets.
5. Uber: Data Mobility & AI-Powered Analytics
Challenge:
- Need to handle massive amounts of real-time GPS, ride request, and transaction data.
- Storage systems needed to support machine learning models for pricing, fraud detection, and demand forecasting.
- Data required high-speed mobility across global data centers and cloud infrastructure.
Solution:
- Built an in-house data lake (Hudi) to improve storage efficiency.
- Implemented multi-region cloud storage (AWS & Google Cloud) for real-time analytics.
- Optimized AI storage pipelines for faster model training and inference.
Outcome:
- Scaled to handle petabytes of data with fast retrieval speeds.
- Enhanced AI-driven pricing and routing models with low-latency storage.
- Improved fraud detection through real-time AI analytics.
Key Takeaways from Enterprise Case Studies:
- Cloud adoption (AWS, Azure, Google Cloud) is a dominant strategy to handle data growth and AI workloads, although the financial implications may make this unsustainable
- Hybrid models (on-prem + cloud) are effective for regulated industries (e.g., finance, healthcare).
- AI-driven storage optimization (edge computing, parallel storage, tiered storage) helps in handling AI/ML workload evolution.
- Data mobility is critical for industries that require real-time processing (e.g., Uber, Tesla).
The Future Outlook for AI
As AI workloads become increasingly central to enterprise operations, organizations must prioritize scalable infrastructure, efficient data management, and optimized inference execution. The next chapter explores the The Future Outlook for AI: 2025 and Beyond, examining the emerging trends, innovations, and strategic directions that will define AI’s role in enterprise success. Businesses that effectively integrate AI-driven workloads into their core operations will position themselves at the forefront of the next digital era.
This article is part of a larger report on AI’s transformative impact on enterprises, infrastructure, and global competitiveness. The full 9 chapter report, “The Enterprise Edge in an AI-Centric World – An Executive Field Guide for 2025” explores the key challenges and opportunities shaping AI adoption. Each chapter provides deep insights into critical aspects of AI deployment, from power constraints and data mobility to automation and geopolitical strategy. Each section, offers actionable recommendations for enterprises, policymakers, and AI infrastructure providers navigating the future of AI.