VP Engineering David Hughes delivers an innovative session at GTC 2025. Stelia presents a session recap.
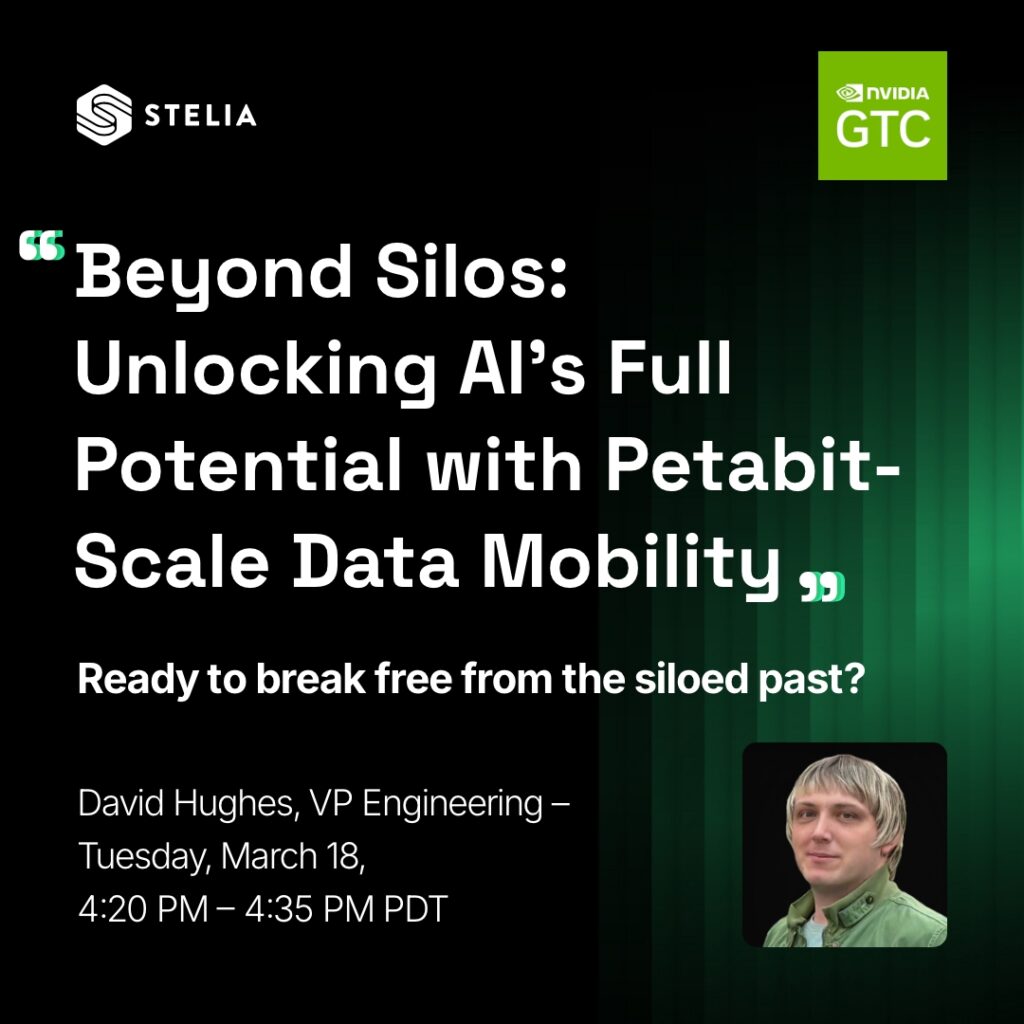
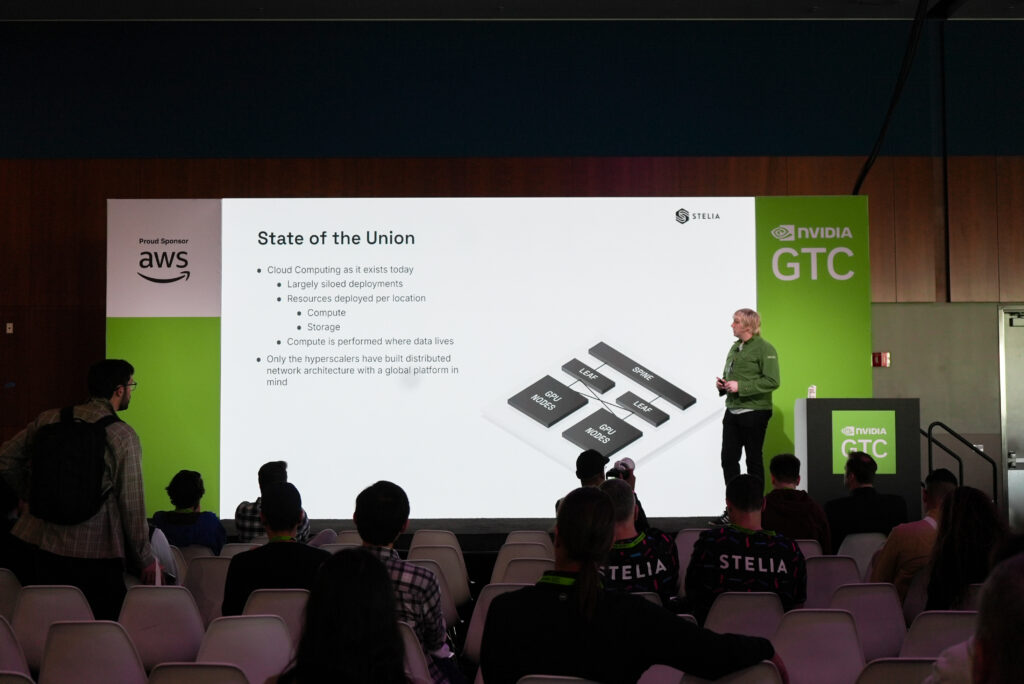
During Tuesday’s in-person session at Nvidia GTC, David Hughes explored why:
- The Cloud Model No Longer Fits AI at Scale
- Siloed Compute is a Bottleneck for AI Workloads
- Data Mobility Unlocks AI Scalability
- Interconnected Elastic Compute is the Future
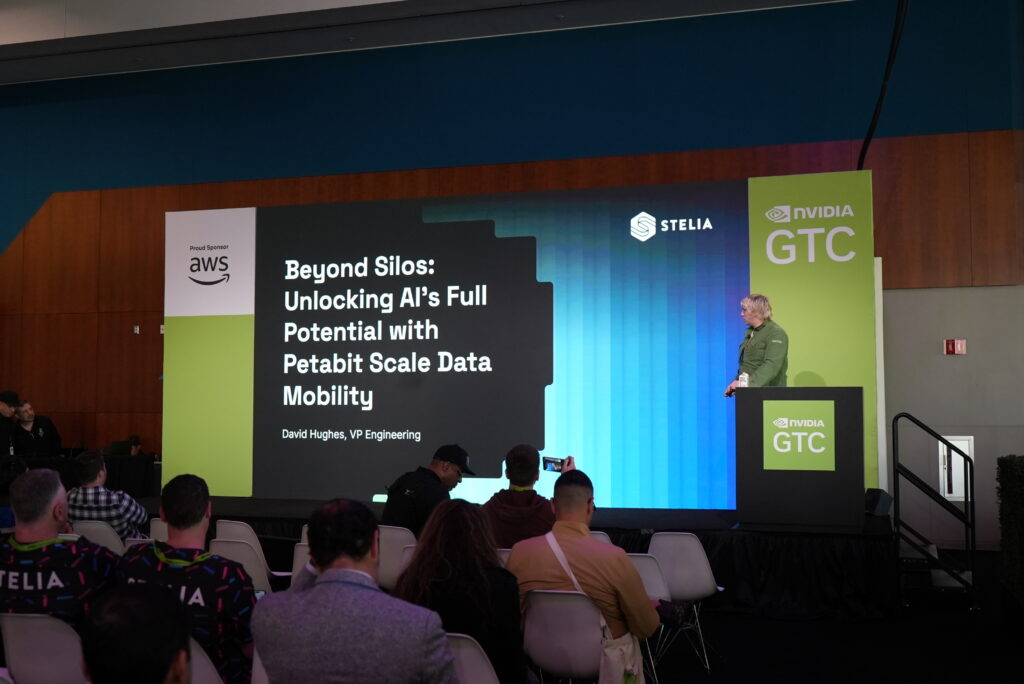
Repeating the Past: GPU-as-a-Service Without a Plan
In the last half-decade, we’ve seen a surge of startups offering GPU-as-a-Service (effectively Infrastructure-as-a-Service). Many of these companies originated from crypto mining, where hardware was simply deployed, run for a fixed period, and then divested. Unfortunately, this short-term mindset doesn’t translate well into a sustainable, multi-tenant cloud model.
Instead of learning from past innovations, these new entrants often:
- Operate like “juiced up 00s web hosts” rather than genuine hyperscalers.
- Deploy individual silos of resources in colocation facilities or old mining sites based on cost alone.
- Fail to design infrastructure for multiple tenants, limiting elasticity and scalability.
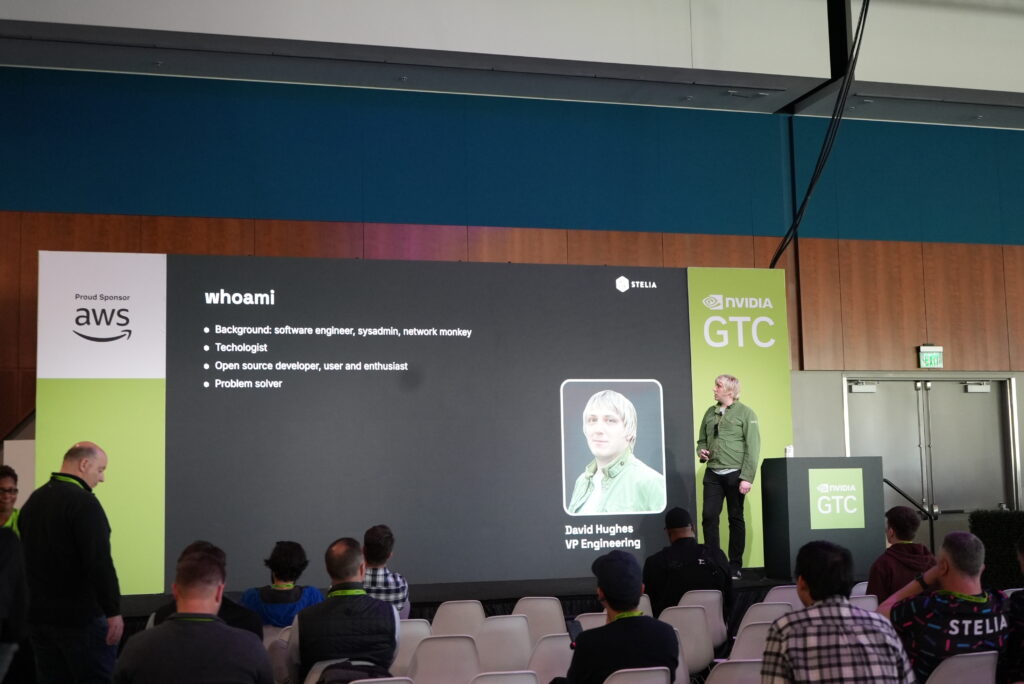
What Hyperscalers Got Right
Starting around 2006, hyperscalers like Facebook faced exponentially growing traffic and resource needs. Out of necessity, they:
- Learned from Past Giants
They acknowledged the mistakes of once-dominant Unix vendors and embraced openness—leveraging open-source technologies like Linux and BSD, rather than reinventing the wheel behind closed doors. - Designed Massive, Multi-Continental Networks
By thinking globally, they ensured traffic could be routed efficiently, preventing bottlenecks and enabling seamless user experiences across continents. - Focused on Elastic, Multi-Tenant Infrastructure
“Single tenant” was never an option at hyperscale. The solution was to engineer platforms capable of dynamically allocating compute, storage, and networking among countless users. - Innovated Collaboratively
Openness became a competitive advantage. Hyperscalers shared white papers, conference presentations, and even hardware designs (e.g., the Open Compute Project), fostering a thriving ecosystem of innovation.
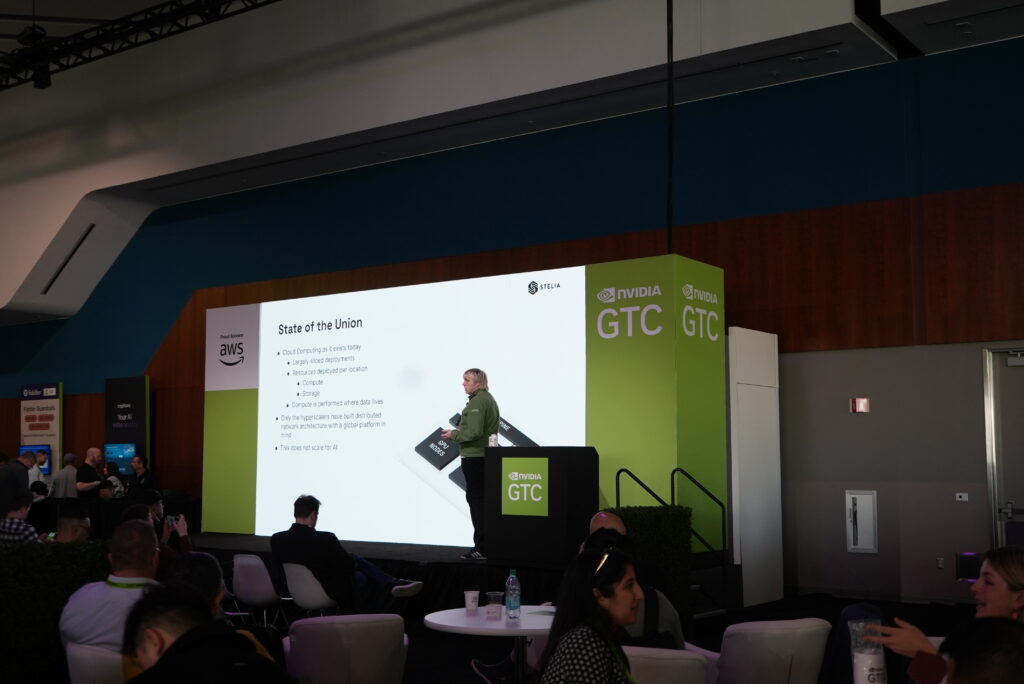
Why the Old Model No Longer Fits AI at Scale
Modern AI workloads aren’t just about running a handful of computations in a single data center. They involve trillions of tokens in model training, require enormous compute resources, and demand global data connectivity. When your AI tasks need to shift data between continents or rapidly spin up GPU clusters on the other side of the world, a siloed, static infrastructure just can’t keep up.
Key Point: The “compute where data resides” model that once defined the cloud now becomes a bottleneck. AI needs elastic resource access across multiple sites, often in real time.
Siloed Compute: The Bottleneck for AI Workloads
- Inefficient Scaling: Training large AI models on isolated clusters forces expensive hardware refreshes and migrations.
- Delayed Innovation: Siloed environments can’t pivot fast enough for rapid prototyping and iteration.
- Restricted Resources: Enterprises, neoclouds, and model builders often outgrow a single data center, leading to slow training times and ballooning costs.
Data Mobility: The New Frontier
What if you could dynamically move data to wherever the compute resources are most available or cost-effective? Enter hyperscale scale data mobility — a platform-level capability that efficiently transfers massive datasets between geographically dispersed locations. This approach:
- Removes Bottlenecks
AI models no longer stall because of data locality constraints. - Scales AI Globally
Training can happen across multiple continents without incurring crippling latency. - Fosters True Elasticity
Resources are allocated on demand, and data moves at petabit-scalespeeds to match AI’s appetite.
Interconnected Elastic Compute Is the Future
When data, compute, and networking operate as a single, fluid ecosystem, AI can truly scale:
- Compute On Demand: Provision GPUs anywhere in the network.
- Global Data Access: Transfer datasets at petabit speeds across oceans and continents.
- Unified Management: Orchestrate compute, storage, and networking through a single control plane.
This isn’t just a wish-list; it’s the next logical step, echoing the spirit of how hyperscalers rose to prominence. The difference now is the sheer scale demanded by AI.
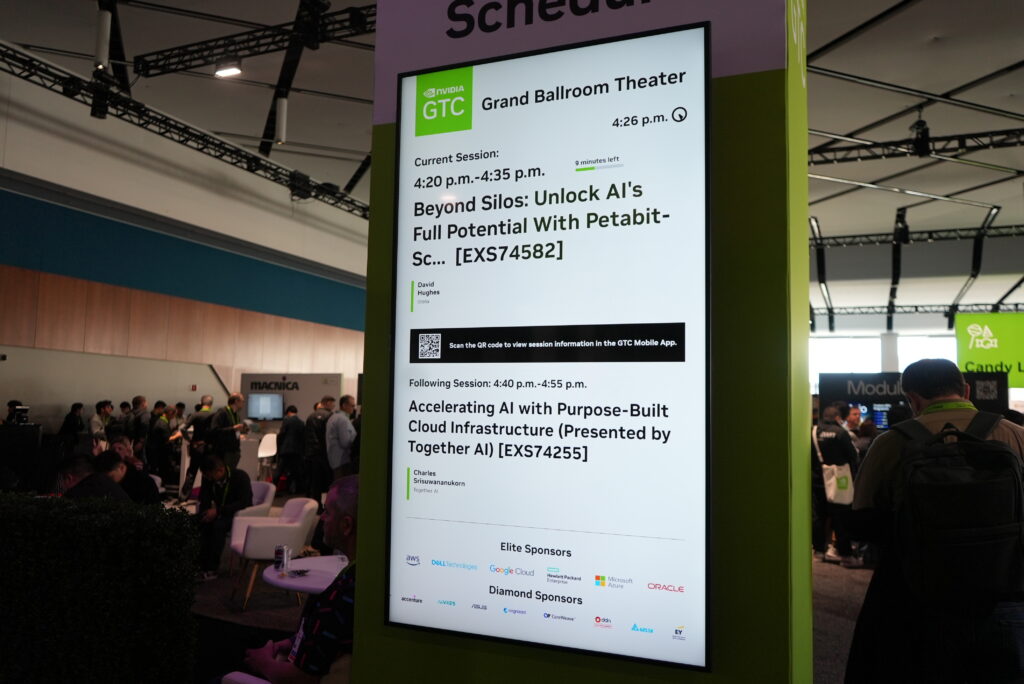
Final Thoughts
The industry has seen too many GPU-as-a-Service ventures trying to reinvent the wheel without honoring the lessons of hyperscalers. Now, as AI workloads become more demanding than ever, it’s time to embrace the future of data mobility and interconnected elasticity. Let’s move beyond silos and step into a world where AI thrives on a truly global, scalable infrastructure.
We hope you enjoyed our in-person session at GTC!
📍 Visit Stelia at Booth #1033 in Expo Hall 3 to see how our intelligence platform optimises AI workloads by dynamically allocating resources – from pre-training to edge inference – globally.
💡 We’re already partnering with the most advanced and ambitious companies where AI is core to their business. Drop by the booth, grab some swag, and see how we can help you too!
For the GTC 2025 presentation deck, please find this below.